Guide to the Perplexed about Math and Macroeconomics
2019-09-24 16:26:24
By Thomas Sargent
Written for SIQEF Newsletter
Download the PDF version of the article here
Q. Some of the best writing in economics is purely “literary” and explicitly uses no mathematics at all. Doesn't that give you second thoughts about some of the things you say above?
A. Give me some examples of such good writers.
Q. Walter Bagehot's “Lombard Street” and Milton Friedman and Anna Schwartz's Monetary History of the United States and John Maynard Keynes's “Tract on Monetary Reform.”
A. Each of those writers had excelled at advanced mathematics as students. If you read their works, you'll see that the authors write clearly about how they reason and how they make inferences from data by combining them with a theory. So, knowing math seems to make you a better writer in natural language too!
Q. Do you think the language of math puts unnecessary restrictions on theory building at times? Some people have made the claim that using math limits the scope and complexity of the models we can build. Or, in order to build a tractable and solvable model, we have to make unrealistic and oversimplified assumptions.
A. The whole point of math is to put restrictions on how we go about building a model. If you can't say it in math, you aren't speaking clearly and explicitly and completely.
If someone thinks that “natural language” is a better tool than math, I recommend that she or he read Stephen Weinberg's account of the history of science in his book “To Explain the World.” Weinberg also explains why “unrealistic” and “oversimplified” assumptions have proved to be of practical use time and again.
Q. Would you say that economics should be considered a natural science or engineering, rather than humanities?
A. An exciting part of modern economics is like engineering. Here I have in mind works on “mechanism design” and “optimal regulation” and on “optimal contracts” and on “optimal monetary and fiscal policy.” I recommend reading the autobiographical essay by Paul Samuelson in the MIT Press book “Lives of the Laureates.” Samuelson explains why mathematical and statistical methods have become so successful and have come to dominate modern economics, and why “literary” methods will not come back. I cannot say these things as well as Samuelson did.
Written for SIQEF Newsletter
Download the PDF version of the article here
Q. Why do working economists today reason in terms of mathematics and present their ideas with mathematics?
A. We use arithmetic because we count things. Also, a fascinating thing about economics is that our job is to describe and understand decisions of and interactions among people who also count things. Some of the things that they count and care about are either identical with or constituents of the things that we count. The people whom we study and we as economists measure prices and quantities and incomes and wealth.
Q. Why do we go beyond arithmetic and use higher mathematics like algebra, geometry, and calculus to analyze data and the people who make the decisions that created the data?
A. We use such higher mathematics because we want to reason coherently. We want to reason explicitly and put all of our cards on the table – meaning that we explicitly state both the “if” and the “then” and the steps that lead from “if” to “then.” Mathematics is the most efficient and universal language in which such reasoning occurs.
Q. Is the coherence of a model that mathematics brings sufficient for the model to be a good one?
A. Definitely no. You can write down a coherent model that describes an artificial world that is purely imaginary.
Q. Then what's so great about coherence?
A. We like it because it means that arguments fit together, and we aren't assuming something in one part of a structure that contradicts something we have assumed in another part. Only with a coherent model can we get a story that sorts out “cause” and “effect.”
I confess that it is an esthetic judgment to say that we like coherence - an act of faith that coherent models have a better chance of helping us understand data than incoherent ones.
Q. What tools are available for checking whether one coherent theoretical model is more realistic than another?
A. Mathematical statistics.
Q. Is that why working research economists use mathematical statistics so pervasively?
A. Yes. And also, because we want to be precise about risk and uncertainty and to state carefully about what we know and what we don't know. We don't know everything.
But just because we don't know everything, it does not follow that we know nothing. Statistics is a tool that allows us to draw lines between what we know and what we don't know.
Q. Aren't data and mathematical statistics enough by themselves? Why do we want to use economic theory too, and to combine economic theory with statistics?
A. Because the message of modern (Bayesian) statistical theory is that all you can learn from data is features or parameters of a model. If you ask the data to “speak for themselves” they will be completely silent. Statistical theory is about learning parameters of a model. And for research macroeconomists, that is a macroeconomic model with people inside it who are making decisions and also “doing their own statistical analyses.”
Q. Are there examples where the methodology of mixing data and theory together in the way that you describe have actually been of practical use?
A. Yes, there are many examples. A good way to answer this question is to remember the origins of “operations research” and “information theory” in the United Kingdom and the United States and the Soviet Union during World War II. The armed forces in these countries faced a number of practical problems that can broadly be classified as “optimal resource allocation problems” (the origin of “operations research”) and code breaking and code creation (the original of “information theory”) that people trained in the liberal arts instead of science could not solve. Intellectual giants such as Milton Friedman, Abraham Wald, Alan Turing, Claude Shannon and others made huge contributions to the allied war effort against the Nazis and Japanese armed forces by applying and inventing tools in optimization theory and what later came to be recognized as Bayesian statistical theory. After the war, some of these same people turned their attention to making Keynesian economics rigorous and scientific by inventing the modern theory of simultaneous equations that could be estimated and used to help monetary and fiscal authorities design good policies quantitatively.
Q. Are there other examples?
A. Many more. A modern example is the development of “algorithmic mechanism design” - a hot topic now that combines information theory and optimal resource allocation and computer programming to design auctions and other exchange platforms. These are developed and used by companies like Alibaba and Tencent and Amazon and others. Another wonderful application is the recent achievement of “alpha go” in using dynamic programming and game theory and computer programming and Monte Carlo simulation to create an artificially intelligent “Go” player that defeated the best human “Go” player in the world!
Q. Don't “artificial intelligence” and “machine learning” employ a different philosophy that contradicts what you just said earlier about the need to combine “theory” and “data”? Can't data themselves teach you what you should know from experience?
A. No.
Q. Are you saying that machine learning is just an application of statistics?
A. If you remove the word “just” then I'll agree with the statement.
Q. If you agree, why do you want to remove the word “just”?
A. Because machine learning has taken advantage of two very important technical improvements that have empowered researchers to use statistics more widely and inexpensively. The two advances are (a) the acquisition of very large and diverse data sets, and (b) the availability of cheap and powerful and big computers.
Q. Why are working macroeconomists like you and the economists at central banks and treasuries preoccupied with developing models that are sufficiently precise that they can be put on a computer and simulated?
A. Getting a model to be explicit enough and in good enough shape to be put on a computer is a key step in comparing a model with data and in learning about the model from the data. And of course, mathematics is the language that computers hear and speak.
So yes, we confess that we want to build models that are sufficiently coherent and precise that they can be expressed as pseudo code that can be handed to a computer programmer who can simulate the model and generate objects that the computer can compare to the objects that we measure.
Q. Can you give an example say in macroeconomics? Is there something that the tools you like can uncover that can’t be uncovered by merely undergraduate macroeconomics?
A. Yes. For example, the models that central banks use to understand the origins of banking panics and how to moderate them. Also, another example, the methods that credit ranking agencies use to assess sovereign debt and to predict likelihood of sovereign debt crises. Also, the quantitative methods that some researchers use to understand the dynamics of international exchange rates quantitatively and how they are affected by monetary and fiscal policies in multiple countries.
Q. Do you regret that the use of mathematics limits our ability to communicate with policy makers and people who are not economists?
A. Would you ask an engineer or a physicist that question?
Q. Do you think it is research economists' responsibility to promote good economic thinking, or explain what good economic decision-making is, to the public?
A. We use arithmetic because we count things. Also, a fascinating thing about economics is that our job is to describe and understand decisions of and interactions among people who also count things. Some of the things that they count and care about are either identical with or constituents of the things that we count. The people whom we study and we as economists measure prices and quantities and incomes and wealth.
Q. Why do we go beyond arithmetic and use higher mathematics like algebra, geometry, and calculus to analyze data and the people who make the decisions that created the data?
A. We use such higher mathematics because we want to reason coherently. We want to reason explicitly and put all of our cards on the table – meaning that we explicitly state both the “if” and the “then” and the steps that lead from “if” to “then.” Mathematics is the most efficient and universal language in which such reasoning occurs.
Q. Is the coherence of a model that mathematics brings sufficient for the model to be a good one?
A. Definitely no. You can write down a coherent model that describes an artificial world that is purely imaginary.
Q. Then what's so great about coherence?
A. We like it because it means that arguments fit together, and we aren't assuming something in one part of a structure that contradicts something we have assumed in another part. Only with a coherent model can we get a story that sorts out “cause” and “effect.”
I confess that it is an esthetic judgment to say that we like coherence - an act of faith that coherent models have a better chance of helping us understand data than incoherent ones.
Q. What tools are available for checking whether one coherent theoretical model is more realistic than another?
A. Mathematical statistics.
Q. Is that why working research economists use mathematical statistics so pervasively?
A. Yes. And also, because we want to be precise about risk and uncertainty and to state carefully about what we know and what we don't know. We don't know everything.
But just because we don't know everything, it does not follow that we know nothing. Statistics is a tool that allows us to draw lines between what we know and what we don't know.
Q. Aren't data and mathematical statistics enough by themselves? Why do we want to use economic theory too, and to combine economic theory with statistics?
A. Because the message of modern (Bayesian) statistical theory is that all you can learn from data is features or parameters of a model. If you ask the data to “speak for themselves” they will be completely silent. Statistical theory is about learning parameters of a model. And for research macroeconomists, that is a macroeconomic model with people inside it who are making decisions and also “doing their own statistical analyses.”
Q. Are there examples where the methodology of mixing data and theory together in the way that you describe have actually been of practical use?
A. Yes, there are many examples. A good way to answer this question is to remember the origins of “operations research” and “information theory” in the United Kingdom and the United States and the Soviet Union during World War II. The armed forces in these countries faced a number of practical problems that can broadly be classified as “optimal resource allocation problems” (the origin of “operations research”) and code breaking and code creation (the original of “information theory”) that people trained in the liberal arts instead of science could not solve. Intellectual giants such as Milton Friedman, Abraham Wald, Alan Turing, Claude Shannon and others made huge contributions to the allied war effort against the Nazis and Japanese armed forces by applying and inventing tools in optimization theory and what later came to be recognized as Bayesian statistical theory. After the war, some of these same people turned their attention to making Keynesian economics rigorous and scientific by inventing the modern theory of simultaneous equations that could be estimated and used to help monetary and fiscal authorities design good policies quantitatively.
Q. Are there other examples?
A. Many more. A modern example is the development of “algorithmic mechanism design” - a hot topic now that combines information theory and optimal resource allocation and computer programming to design auctions and other exchange platforms. These are developed and used by companies like Alibaba and Tencent and Amazon and others. Another wonderful application is the recent achievement of “alpha go” in using dynamic programming and game theory and computer programming and Monte Carlo simulation to create an artificially intelligent “Go” player that defeated the best human “Go” player in the world!
Q. Don't “artificial intelligence” and “machine learning” employ a different philosophy that contradicts what you just said earlier about the need to combine “theory” and “data”? Can't data themselves teach you what you should know from experience?
A. No.
Q. Are you saying that machine learning is just an application of statistics?
A. If you remove the word “just” then I'll agree with the statement.
Q. If you agree, why do you want to remove the word “just”?
A. Because machine learning has taken advantage of two very important technical improvements that have empowered researchers to use statistics more widely and inexpensively. The two advances are (a) the acquisition of very large and diverse data sets, and (b) the availability of cheap and powerful and big computers.
Q. Why are working macroeconomists like you and the economists at central banks and treasuries preoccupied with developing models that are sufficiently precise that they can be put on a computer and simulated?
A. Getting a model to be explicit enough and in good enough shape to be put on a computer is a key step in comparing a model with data and in learning about the model from the data. And of course, mathematics is the language that computers hear and speak.
So yes, we confess that we want to build models that are sufficiently coherent and precise that they can be expressed as pseudo code that can be handed to a computer programmer who can simulate the model and generate objects that the computer can compare to the objects that we measure.
Q. Can you give an example say in macroeconomics? Is there something that the tools you like can uncover that can’t be uncovered by merely undergraduate macroeconomics?
A. Yes. For example, the models that central banks use to understand the origins of banking panics and how to moderate them. Also, another example, the methods that credit ranking agencies use to assess sovereign debt and to predict likelihood of sovereign debt crises. Also, the quantitative methods that some researchers use to understand the dynamics of international exchange rates quantitatively and how they are affected by monetary and fiscal policies in multiple countries.
Q. Do you regret that the use of mathematics limits our ability to communicate with policy makers and people who are not economists?
A. Would you ask an engineer or a physicist that question?
Q. Do you think it is research economists' responsibility to promote good economic thinking, or explain what good economic decision-making is, to the public?
A. Yes, and it can be challenging because economics is technical and quantitative, and some members of the public and public officials are less comfortable than others are in appreciating economics. It is important to present things as nontechnically and simply as possible, but at the same time not to oversimplify and to not to neglect important qualifications and tradeoffs. Some of the giants in economics have been very good at expressing key ideas nontechnically, for example Adam Smith and Alfred Marshall. They were masters of logic and language. These are rare skills that I admire very much when I see them in others.
Q. Some of the best writing in economics is purely “literary” and explicitly uses no mathematics at all. Doesn't that give you second thoughts about some of the things you say above?
A. Give me some examples of such good writers.
Q. Walter Bagehot's “Lombard Street” and Milton Friedman and Anna Schwartz's Monetary History of the United States and John Maynard Keynes's “Tract on Monetary Reform.”
A. Each of those writers had excelled at advanced mathematics as students. If you read their works, you'll see that the authors write clearly about how they reason and how they make inferences from data by combining them with a theory. So, knowing math seems to make you a better writer in natural language too!
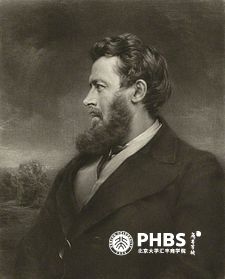
Walter Bagehot, editor of “The Economist” and author of “The English Constitution” and “Lombard Street.”
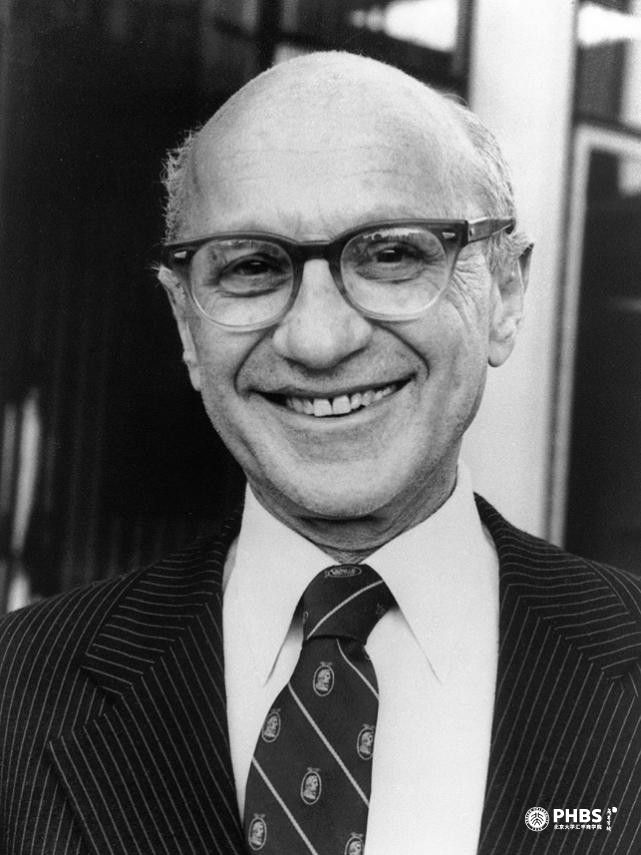
Milton Friedman, 1976 Nobel laureate in Economics.
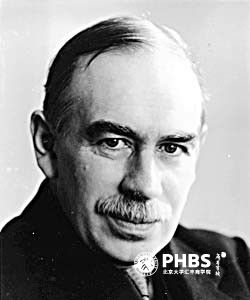
John Maynard Keynes.
Q. Do you think the language of math puts unnecessary restrictions on theory building at times? Some people have made the claim that using math limits the scope and complexity of the models we can build. Or, in order to build a tractable and solvable model, we have to make unrealistic and oversimplified assumptions.
A. The whole point of math is to put restrictions on how we go about building a model. If you can't say it in math, you aren't speaking clearly and explicitly and completely.
If someone thinks that “natural language” is a better tool than math, I recommend that she or he read Stephen Weinberg's account of the history of science in his book “To Explain the World.” Weinberg also explains why “unrealistic” and “oversimplified” assumptions have proved to be of practical use time and again.
Q. Would you say that economics should be considered a natural science or engineering, rather than humanities?
A. An exciting part of modern economics is like engineering. Here I have in mind works on “mechanism design” and “optimal regulation” and on “optimal contracts” and on “optimal monetary and fiscal policy.” I recommend reading the autobiographical essay by Paul Samuelson in the MIT Press book “Lives of the Laureates.” Samuelson explains why mathematical and statistical methods have become so successful and have come to dominate modern economics, and why “literary” methods will not come back. I cannot say these things as well as Samuelson did.
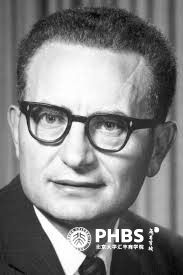
Paul Samuelson, 1970 Nobel Laureate in Economics and a founder of mathematical economics.
Get involved with your College and see what events and opportunities are available.
For a full listing of University events open to the public, visit the What's On website.
Share this